Public Lectures on Data Science and Machine Learning
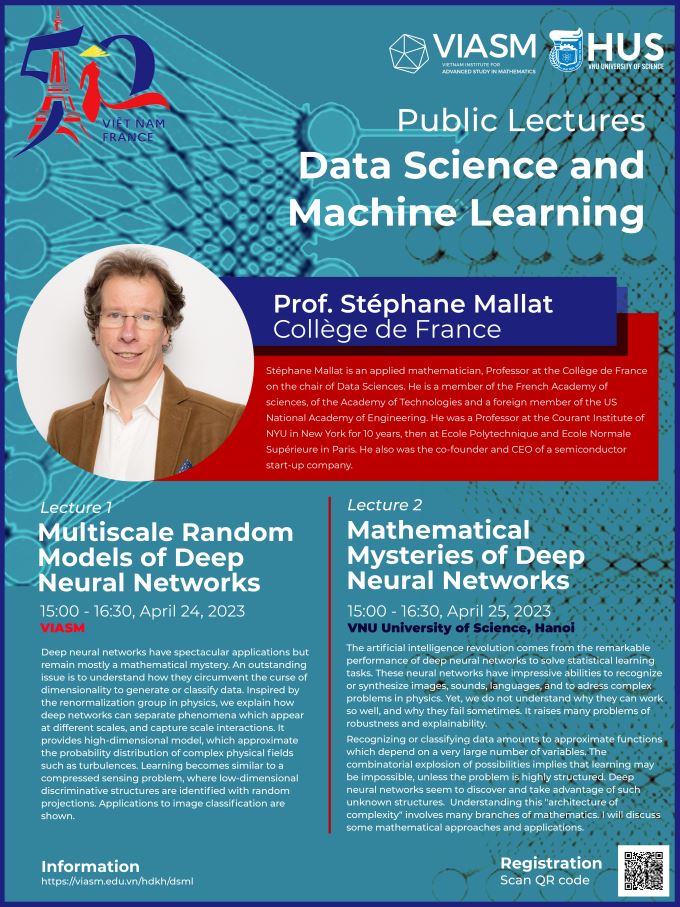
Thời gian: 15:00 đến 16:30 Ngày 24/04/2023
Địa điểm: VIASM & VNU-HUS
Speaker: Stéphane Mallat, Collège de France
Bio:
Stéphane Mallat is an applied mathematician, Professor at the Collège de France on the chair of Data Sciences. He is a member of the French Academy of sciences, of the Academy of Technologies and a foreign member of the US National Academy of Engineering. He was a Professor at the Courant Institute of NYU in New York for 10 years, then at Ecole Polytechnique and Ecole Normale Supérieure in Paris. He also was the co-founder and CEO of a semiconductor start-up company.
Stéphane Mallat received many prizes for his research in machine learning, signal processing and harmonic analysis. He developed the multiresolution wavelet theory and algorithms at the origin of the compression standard JPEG-2000, and sparse signal representations in dictionaries through matching pursuits. He currently works on mathematical models of deep neural networks, for data analysis and physics.
******************************
Registration: Here
******************************
Multiscale Random Models of Deep Neural Networks
Time: 15:00-16:30, 24/4/2023
Venue: Vietnam Institute for Advanced Study in Mathematics (VIASM)
Deep neural networks have spectacular applications but remain mostly a mathematical mystery. An outstanding issue is to understand how they circumvent the curse of dimensionality to generate or classify data. Inspired by the renormalization group in physics, we explain how deep networks can separate phenomena which appear at different scales, and capture scale interactions. It provides high-dimensional model, which approximate the probability distribution of complex physical fields such as turbulences. Learning becomes similar to a compressed sensing problem, where low-dimensional discriminative structures are identified with random projections. Applications to image classification are shown.
******************************
Mathematical Mysteries of Deep Neural Networks
Time: 15:00-16:30, 25/4/2023
Venue: VNU University of Science (VNU-HUS)
The artificial intelligence revolution comes from the remarkable performance of deep neural networks to solve statistical learning tasks. These neural networks have impressive abilities to recognize or synthesize images, sounds, languages, and to adress complex problems in physics. Yet, we do not understand why they can work so well, and why they fail sometimes. It raises many problems of robustness and explainability.
Recognizing or classifying data amounts to approximate functions which depend on a very large number of variables. The combinatorial explosion of possibilities implies that learning may be impossible, unless the problem is highly structured. Deep neural networks seem to discover and take advantage of such unknown structures. Understanding this "architecture of complexity" involves many branches of mathematics. I will discuss some mathematical approaches and applications.